The presence of a huge number of machines in industrial automation factories and the elevated cost of downtime, produce large expenses for production line maintenance. Getting a more accurate evaluation of robot performance helps to avoid damaging the production capacity contingently by 5 to 20 % in certain cases[1].
But collecting data from different factories to build powerful AI tools can raise privacy issues. Data used to train AI models can be sensitive company data but also lead to personal data concerns, for instance data include information about operators working at the plant.
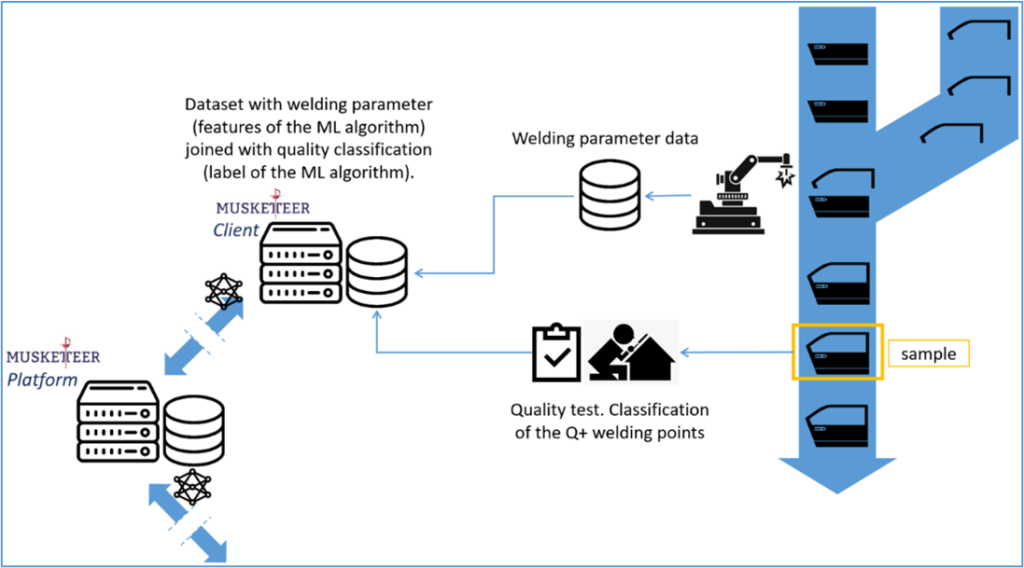
Using the MUSKETEER platform provides a robust solution mixing a federated machine learning approach (local training using the Musketeer client) with privacy-preserving technologies (highly customized encryption, data poisoning attacks mitigation) while respecting the sovereignty of the users based on IDSA concepts.
The IDS reference architecture is the de facto standard for creating and operating data ecosystems. Its approach is to ensure data sovereignty by facilitating the secure exchange of data between trusted parties. Certified participants are granted access to the data ecosystem, in which they can attach usage policies to their data before they make it available to other participants.
The Musketeer client acting as a potential IDS connector and the overall ecosystem is currently assessing the proper compliance with the IDSA standard[2]. This approach has been successfully tested in a manufacturing use case helping to solve complex challenges for the automotive sector:
- Improving the welding quality assessment to develop predictive maintenance for robots while increasing product safety at the same time.
- Training a welding quality assessment algorithm on large datasets from multiple factories
The project has received funding from the European Horizon 2020 research and innovation program under grant agreement No. 824988.
To find out more about this story and discover the complete scenario along with user testimonials, visit: https://musketeer.eu/publications/
Sources
[1] https://www2.deloitte.com/content/dam/Deloitte/us/Documents/process and operations/us cons predictive maintenance.pdf
[2] https://www.internationaldataspaces.org/wp content/uploads/2019/03/IDS Reference Architecture Model 3.0.pdf