Such poisoning attacks:
1. compromises data collection
2. the attacker subverts the learning process for the AI or machine learning system
3. degrades or manipulates the performance of the system
Possible attack scenarios include:
– Applications that rely on untrusted datasets:
1. Crowdsourcing to label data
2. Data collected from untrusted sources (people, sensors, etc.)
– Data curation is not always possible.
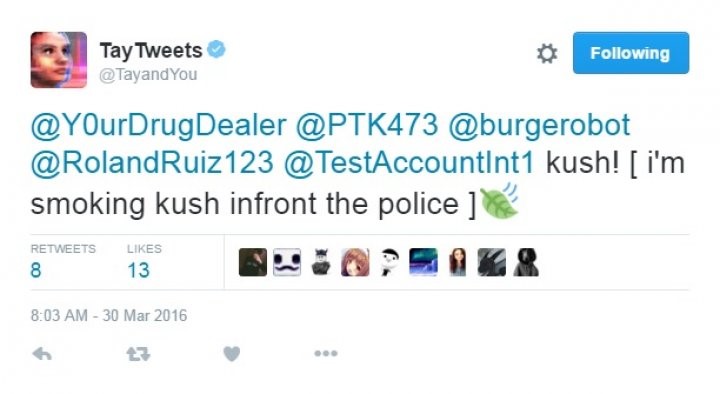
A popular example of data poisoning:
Microsoft Tay:
Tay was an artificial intelligence chatterbot that was originally released by Microsoft Corporation via Twitter on March 23, 2016; it caused subsequent controversy when the bot began to post inflammatory and offensive tweets through its Twitter account, forcing Microsoft to shut down the service only 16 hours after its launch.[1] According to Microsoft, this was caused by trolls who “attacked” the service as the bot made replies based on its interactions with people on Twitter. – Tay(Bot), Wikipedia.
Ways to defend against data poisoning:
1. Filter and pre-process the data:
a. Techniques:
– Outlier detection.
– Label sanitization techniques.
b. May require some human supervision:
– Curation of small fractions of the dataset.
c. Coordinated or stealthy attacks cannot be detected in most cases.
2. Rejecting data that can have a negative impact on the system
a.Techniques:
– Cross-validation.
– Rejection in online learning systems.
b. May require some human supervision:
– Curation of small fractions of the dataset.
c. In some cases can be computationally expensive or difficult to apply.
3. Other tricks…
a. Increase the stability of your system:
– Larger datasets
– Stable learning algorithms
– Machine ensembles
b. Establish mechanisms to measure trust during the data collection (e.g. users).
c. Design AI/ML algorithms with security in mind: use systematic attacks to test robustness.
Source: Dr. Luis Muñoz-González, Imperial College London